The Dynamic Interplay of AI and Cybersecurity: Uncovering Opportunities and Navigating Risks
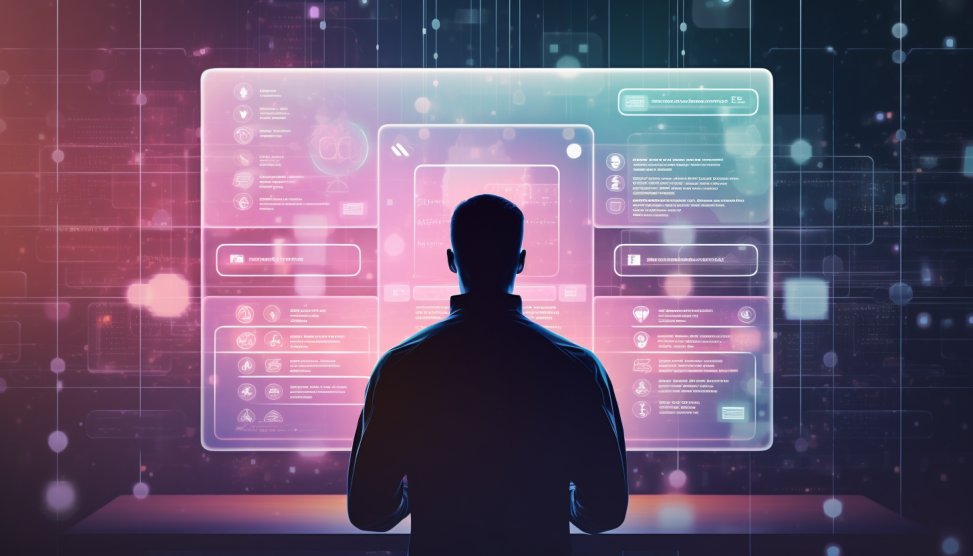
The fusion of AI and cybersecurity is reshaping how organizations protect their digital assets. By leveraging AI and machine learning in cybersecurity, we've entered an era where we can detect and counteract threats with a precision that was previously unimaginable.
Yet, the capabilities of AI come with their own set of intricacies. While it offers enhanced protection, it also presents new challenges requiring careful navigation to ensure data privacy and system integrity.
This article will explore the significance of adopting AI in cybersecurity, its benefits, potential pitfalls, and strategies for effective implementation.
How is AI Different from Traditional Cybersecurity Approaches?
Traditional cybersecurity approaches largely rely on predefined rules, signatures, and historical threat data to detect and counteract cyber threats. While effective against known threats, these methods often struggle to identify novel or rapidly evolving cyber-attacks. AI, on the other hand, introduces a dynamic and adaptive approach to cybersecurity.
Using machine learning, AI systems can analyze vast amounts of data, identify patterns, and make real-time data-driven decisions. Unlike traditional systems that are past-centric and built around known attacks, AI can predict potential threats, recognize anomalies, and adapt its defenses based on new information. This proactive stance allows AI to detect and respond to threats with greater accuracy and speed than conventional methods.
Additionally, AI can automate repetitive tasks, easing the burden on security professionals and ensuring a more comprehensive and timely response to potential security breaches. While traditional cybersecurity is reactive and rule-based, AI offers a proactive, adaptive, and data-driven approach to safeguarding digital assets.
How AI has Changed the Cybersecurity Landscape
The integration of AI into the cybersecurity domain has not only revolutionized defense mechanisms but has also provided businesses with a proactive approach to handling cyber threats. This transformative technology has been a game-changer, offering both opportunities and challenges in the digital realm.
AI-Powered Attacks
While there's a growing concern about the potential of AI being harnessed by cyber criminals, it's essential to understand that the same technology is being employed to counteract these threats. Advanced AI algorithms are being developed to detect and neutralize AI-driven malicious activities.
Cybersecurity professionals can devise strategies that anticipate and counteract these threats by understanding the patterns and methodologies of AI-powered attacks, ensuring a dynamic defense mechanism.
Advanced Threat Detection
AI's capability for advanced threat detection stands out as one of its most significant contributions to cybersecurity. Machine learning models, trained on vast datasets, can sift through enormous amounts of data in real time, identifying patterns and anomalies that might elude human analysts or conventional systems.
These AI-driven systems predict potential threats and adapt to evolving threat vectors, ensuring that businesses are reactive and proactive in their cybersecurity approach.
Behavioral Biometrics and Authentication
Beyond the traditional authentication methods, AI introduces behavioral biometrics, a sophisticated way of ensuring user authenticity. By continuously analyzing and learning from user behaviors, such as keystroke patterns, mouse movements, and even browsing habits, AI can create a unique profile for each user.
This means that even if a malicious user gets hold of login credentials, the system can detect any deviation from the established behavioral profile, adding an extra layer of security.
Automated Incident Response
When a cyber incident occurs, the speed and efficiency of the response can make all the difference. AI-driven systems enhance this response time by automating many of its aspects. Upon detecting a breach, these systems can immediately isolate affected segments, initiate predefined security protocols, notify relevant personnel, and even suggest mitigation strategies.
This reduces the potential damage and ensures that recovery efforts are swift and efficient.
How Can AI be Used for Cybersecurity Management?
By leveraging AI's unparalleled computational capabilities, businesses can now anticipate, analyze, and act upon cyber threats with unprecedented precision and efficiency. Here are just a few examples:
Quick Anomaly Detection
One of AI's standout capabilities is its ability to rapidly detect anomalies in vast datasets. By establishing a "normal" network behavior baseline, AI can instantly spot deviations, whether an unusual data transfer or an unauthorized access attempt.
This rapid detection ensures that potential threats are identified and neutralized before they can inflict significant damage.
User Authentication and Access Control
AI enhances traditional authentication methods by introducing dynamic and adaptive access controls. By continuously learning from user behaviors, AI can develop a comprehensive profile for each user, ensuring that any deviation from this profile, like a sudden change in location or device, triggers additional authentication measures, thereby adding an extra layer of security.
Cybersecurity Predictive Analytics
AI's predictive analytics capabilities allow businesses to forecast potential cyber threats. By analyzing past incidents and current network behavior, AI can provide insights into potential future threats and vulnerabilities, allowing companies to take preemptive measures and fortify their defenses.
Security Vulnerability Assessments
AI-driven systems can conduct comprehensive security assessments, scanning networks for vulnerabilities malicious actors might exploit. These assessments are not just limited to known vulnerabilities but also predict potential future weak points, ensuring that businesses are always a step ahead in their cybersecurity efforts.
What are the Potential Risks of Using AI for Cybersecurity?
With all its merits in cybersecurity enhancement, it’s important to consider the challenges and vulnerabilities that also come with AI integration:
Adversarial Attacks
These sophisticated techniques involve cybercriminals subtly manipulating data to deceive AI models. By introducing data that appears normal but is slightly altered, attackers can cause AI systems to misinterpret the input, leading to incorrect decisions.
This can allow malicious activities to go undetected or even manipulate the AI into taking unintended actions.
Data Poisoning
This tactic involves introducing malicious data into the AI's training set, effectively "teaching" it incorrect behaviors. When trained on this skewed data, an AI system can make erroneous judgments, potentially misclassifying harmful activities as safe or vice versa.
Model Inversion Attacks
Here, cybercriminals attempt to reverse-engineer the AI's decision-making process. By analyzing the outputs of a machine learning model, they can deduce information about its training data, potentially revealing sensitive information or proprietary algorithms.
Overreliance and Blind Spots
While AI's ability to process vast datasets is unparalleled, solely depending on it can lead to vulnerabilities. AI models trained on past data might not recognize new, innovative attack vectors. This overreliance can lead to security blind spots, where novel threats go undetected.
Privacy Concerns
AI's insatiable appetite for data can sometimes infringe on user privacy. There's a risk of data misuse, especially if the AI system is compromised or lacks stringent data governance policies. This can lead to unauthorized data access or breaches, jeopardizing user trust.
Lack of Explainability
One of the significant challenges with deep learning AI models is their inherent opacity. Their decision-making processes can be intricate and hard to decipher. This "black box" nature can be problematic when trying to diagnose false positives or understand the rationale behind specific security decisions.
In essence, while AI's capabilities in cybersecurity are groundbreaking, it's crucial to be clear of its potential pitfalls. Balancing its strengths with a thorough understanding of its vulnerabilities ensures that organizations can harness AI's power effectively while maintaining robust security protocols.
Famous Use Cases of AI in Cybersecurity
Several high-profile companies are leading the charge in cybersecurity AI integration. For example, Cisco uses AI to enhance its threat detection capabilities, with its 2018 Security Capabilities Benchmark Study revealing that a significant portion of organizations rely on automation, machine learning, and AI for cyber threat detection.
Darktrace, another major player in the cybersecurity arena, employs machine learning to detect, respond to, and mitigate cyber threats in real-time, offering a self-learning system that adapts to new challenges.
With its Magnifier behavioral analytics solution, Palo Alto Networks uses AI to quickly and accurately distinguish between legitimate and malicious activities, ensuring timely threat detection.
IBM's Watson for Cyber Security sifts through vast amounts of data to identify threats, drawing on its extensive knowledge base to provide insights that human analysts might overlook.
Additionally, Google has incorporated AI into its security strategy, using machine learning algorithms to detect phishing emails in Gmail with a reported 99.9% accuracy.
These real-world applications underscore AI's pivotal role in modern cybersecurity, showcasing its potential to revolutionize the industry.
Future of Using AI for Cybersecurity
The trajectory of AI's integration into cybersecurity paints a promising picture for the future. As businesses continue to digitize and the volume of data exponentially grows, the demand for advanced, automated threat detection and response systems will surge.
AI's capability to predict and swiftly identify novel threats, as showcased by companies like Cisco and Darktrace, will become even more crucial. The evolution of machine learning models will lead to more proactive and adaptive security measures capable of anticipating attack vectors before they manifest.
However, as AI systems become more sophisticated, so will cybercriminals, potentially leveraging AI to orchestrate more complex attacks. This underscores the importance of continuous advancements in AI-driven cybersecurity solutions. Furthermore, integrating AI with other emerging technologies, such as quantum computing, could redefine cybersecurity paradigms.
The future will likely witness a dynamic interplay between AI-enhanced cybersecurity measures and the ever-evolving tactics of cyber adversaries, making the role of AI indispensable in the digital defense landscape.
Bottom Line
As AI intertwines with cybersecurity, it's revolutionizing the protective measures organizations employ for their digital resources. With the integration of AI and machine learning, we're now equipped to foresee, pinpoint, and mitigate threats with an accuracy that was once thought unattainable.
However, the capabilities of AI bring both advantages and complications. It amplifies our defense mechanisms but also ushers in new challenges that demand careful oversight to uphold data security and system stability.